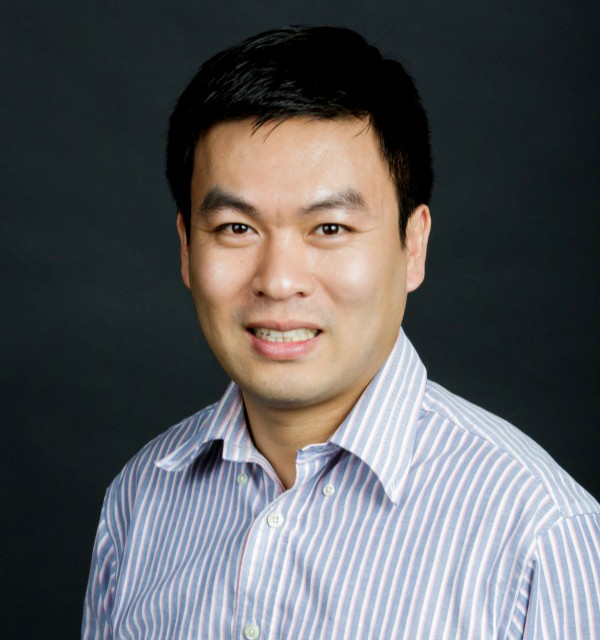
Yong Chen
Dr. Yong Chen, Professor of Biostatistics, founded and directs the Computing, Inference, and Learning Lab (PENNCIL) at University of Pennsylvania. The mission of PENNCIL lab is to develop computational methods and software to transform real-world data into insights, to disseminate the methods and knowledge to research communities, and to bridge the gap from data to actionable health care.
Research areas:
- Real-world data; clinical evidence generation; learning health system; healthcare delivery.
Education:
- Ph.D. in Biostatistics at the Bloomberg School of Public Health, the Johns Hopkins University
- Thesis Advisors: Professor Kung-Yee Liang and Professor Charles Rohde.
- M.A. in Mathematics at the Department of Mathematics, the Johns Hopkins University
- B.S. in Mathematics at the University of Science and Technology of China
Awards:
- 2023. Elected Fellow, the American College of Medical Informatics (ACMI).
- 2023. Best of Annals of Applied Statistics (to be presented at JSM 2023): PALM: Patient-centered Treatment Ranking via Large-scale Multivariate Network Meta-analysis.
- 2022. Winner of the Best Paper in Biometrics by an IBS Member Award: Testing small study effects in multivariate meta-analysis. Biometrics, 76(4), 1240-1250. 2020.
- 2022. Best of Annals of Applied Statistics: Monitoring vaccine safety by studying temporal variation of adverse events using vaccine adverse event reporting system.
- 2021. The Observational Health Data Sciences and Informatics (OHDSI) Titan Award for Methodological Research to recognize extraordinary contributions by an individual, organization, or team in development or evaluation in analytical methods for clinical characterization, population-level effect estimation, or patient-level prediction
- 2021. Best paper award by the Translational Bioinformatics Year-in-Review by American Medical Informatics Association (top 25 papers among 206 papers published in Jan 2020 – March 2021)
- 2020. Elected Fellow, American Statistical Association
- 2019. Distinguished Faculty member at the Department of Biostatistics, Epidemiology and Informatics, the Perelman School of Medicine, University of Pennsylvania
- 2018. Best paper award by the International Medical Informatics Association (IMIA) Yearbook Section on Clinical Research Informatics
- 2018. Elected Member, International Statistical Institute
- 2018. Elected Member, Society for Research Synthesis Methodology
- 2015. Institute of Mathematical Statistics IMS Travel Award
- 2010. Margaret Merrell Award for excellence in research, Department of Biostatistics, The Johns Hopkins University.
- 2005 — 2010. Sommer Scholar, the Bloomberg School of Public Health, the Johns Hopkins University – Dean Alfred Sommer’s leadership training program for the next generation of public health leaders at the Bloomberg School of Public Health, the Johns Hopkins University
- The inaugural class of Hopkins Sommer Scholars in 2005
Selected Publications:
- Selected Statistical Papers:
- Wang, Ye and Chen. Likelihood-based Inference under Non-Convex Boundary Constraints. 2023 Biometrika (in press)
- R Bai, MR Boland, Y Chen. Scalable high-dimensional Bayesian varying coefficient models with unknown within-subject covariance. 2023. Journal of Machine Learning Research 24, 1-49
- Duan, R, Liang, J, Shaw, P, Tang, CY and Chen, Y. 2023 Testing the missing at random assumption in generalized linear models in the presence of instrumental variables. Scandinavian Journal of Statistics. 1–21.https://doi.org/10.1111/sjos.12685
- Duan, R, Ning, Y and Chen, Y. Heterogeneity-aware communication-efficient distributed statistical inference, Biometrika 109.1 (2022): 67-83.
- Bai, R, Moran, G, Antonelli, J, Chen, Y, and Boland, M. Spike-and-Slab Group Lassos for Grouped Regression and Sparse Generalized Additive Models. Journal of the American Statistical Association 117.537 (2022): 184-197.
- Huang, J, Ning, Y, Reid, N and Chen, Y, On specification tests for composite likelihood inference, Biometrika 107.4 (2020): 907-917.
- Huang, J, Ning, Y, Liang, K-Y, and Chen, Y, Composite likelihood inference under boundary conditions, Statistica Sinica 30.2 (2020): 1005-1025.
- Shen, W, Liu, S, Chen, Y, and Ning, J. Regression analysis of longitudinal data with outcome-dependent sampling and informative censoring. Scandinavian Journal of Statistics 46.3 (2019): 831-847.
- Chen, Y, Huang, J, Ning, Y, Liang, K-Y, and Lindsay, B. A conditional composite likelihood ratio test with boundary constraints. Biometrika 105.1 (2018): 225-232.
- Hong, C, Ning, Y, Wang, S, Wu, H, Carroll, RJ and Chen, Y. PLEMT: A novel pseudolikelihood-based EM test for homogeneity in generalized exponential tilt mixture models, Journal of the American Statistical Association 112.520 (2017): 1393–1404.
- Chen, Y, Ning, J, Ning, Y, Liang, K-Y and Bandeen-Roche, K. (2017) On pseudolikelihood inference for semiparametric models with boundary problems. Biometrika, 104 (1): 165–179.
- Ning, J, Chen, Y, Cai, C, Huang, X and Wang, MC. (2015) On the Dependence Structure of Bivariate Recurrent Event Processes: Inference and Estimation, Biometrika 102(2): 345-358.
- Ning, Y, and Chen, Y. (2015) A class of pseudolikelihood ratio tests for homogeneity in exponential tilt mixture models, Scandinavian Journal of Statistics 42 (2), 504–517.
- Chen, Y, and Liang, KY. (2010) On the asymptotic behavior of the pseudolikelihood ratio test statistic with boundary problems, Biometrika, 97 (3), 603–620.
- Selected Biostatistics Papers:
- Luo, C, Duan, R, Edmondson, M, Shi, J, Maltenfort, M, Morris, J, Forrest, C, Hubbard, R, and Chen, Y. (2023) Distributed Proportional Likelihood Ratio Model with Application to Data Integration across Clinical Sites. The Annals of Applied Statistics, (in press)
- Duan, R, Tong, J, Lin, L, Levine, L, Sammel, M, Stoddard, J, Li, T, Schmid, C, Chu, H and Chen, Y. PALM: Patient-centered Treatment Ranking via Large-scale Multivariate Network Meta-analysis, The Annals of Applied Statistics (June 2022).
- Marks-Anglin, A, Luo, C, Piao, J, Gibbons, M, Schmid, C, Ning, J and Chen, Y. EMBRACE: an EM-based Bias Reduction Approach through Copas-Model Estimation for Quantifying the evidence of selective publishing in network meta-analysis, Biometrics 78.2 (2022): 754-765.
- Lian, X, Zhang, J, Hodges, J, Chen, Y, and Chu, H. Accounting for Post-randomization Variables in Meta-analysis: A Joint Meta-Regression Approach, Biometrics (Sep 2021).
- Huang, J, Cai, Y, Du, J., Li, R., Ellenberg, S.S., Hennessy, S., Tao, C., and Chen, Y. Monitoring vaccine safety by studying temporal variation of adverse events using vaccine adverse event reporting system. The Annals of Applied Statistics 2021, 15.1 (2021): 252-269.
- Hong, C, Salanti, G, Morton, S, Riley, R, Chu, H, Kimmel, S, and Chen, Y. Testing small study effects in multivariate meta-analysis, Biometrics 76.4 (2020): 1240-1250.
- Ning, J, Cai, C, Chen, Y, Huang, X and Wang, MC. Semiparametric Modelling and Estimation of Covariate-Adjusted Dependence between Bivariate Recurrent Events, Biometrics 76.4 (2020): 1229-1239.
- Duan, R, Ning, Y, Wang, S, Lindsay, B, Carroll, R, and Chen, Y. A fast score test for generalized mixture models, Biometrics 76.3 (2020): 811-820.
- Wang, L, Chai, X, Chen, Y, and Chen, J. Novel Two-Phase Sampling Designs for Studying Binary Outcomes, Biometrics 76.1 (2020): 210-223.
- Duan, R, Cao, M, Ning, Y, Zhu, M, Zhang, B, McDermott, A, Chu, H, Zhou, X, Moore, J, Ibrahim, J, Scharfstein, D and Chen, Y. Global identifiability of latent class models with applications to diagnostic test accuracy studies: a Grobner basis approach, Biometrics 76.1 (2020): 98-108.
- Ma, X, Lian, X, Chu, H, Ibrahim, J, and Chen, Y. A Bayesian hierarchical model for network meta-analysis of multiple diagnostic tests, Biostatistics, 19.1 (2018): 87–102.
- Hong, C, Ning, Y, Wei, P, Cao, Y and Chen, Y. A semiparametric model for vQTL mapping, Biometrics 73.2 (2017) : 571–581.
- Ning, J, Chen, Y and Piao, J. Maximum likelihood estimation and EM algorithm of Copas-like selection model for publication bias correction. Biostatistics, 18.3 (2017): 495–504.
- Liu, Y, Chen, Y and Chu H. A unification of models for meta-analysis of diagnostic accuracy studies without a gold standard, Biometrics, 71.2 (2017): 538–47.
- Selected Medical Informatics Papers:
- Tong, J, Luo, C, Islam, M, Sheils, N, Buresh, J, Edmondson, M, Merkel, P, Lautenbach, E, Duan, R and Chen, Y. An efficient distributed algorithm with application to COVID-19 data from heterogeneous clinical sites. Nature Partner Journal (NPJ) Digital Medicine, 5, 76 (2022).
- Liu, XK, Duan, R, Luo, C, Ogdie, A, Moore, J, Kranzler, H, Bian, J, and Chen, Y. ADAP: multisite learning with high-dimensional heterogeneous data via A Distributed Algorithm for Penalized regression. Scientific Reports, 12.1 (2022): 1-12.
- Luo, C., Du, J, Butler, A, Cuker, A, Lautenbach, E, Asch, D, Poland, G, Tao, C and Chen, Y. Comparability of clinical trials and spontaneous reporting data regarding COVID-19 vaccine safety, Scientific Reports, 12.1 (2022): 1-8.
- Luo, C., Islam, M.N., Sheils, N.E., Reps, J.M., Buresh, J., Schuemie, M.J., Doshi, J, Werner, R, Asch, D and Chen, Y. dPQL: a lossless distributed algorithm for generalized linear mixed model with application to privacy-preserving hospital profiling. Journal of the American Medical Informatics Association, 29.8 (2022): 1366-1371.
- Edmondson, M, Luo, C, Islam, N, Sheils, N, Buresh, J, Chen, Z, Bian, J, and Chen, Y. Distributed Quasi-Poisson Regression Algorithm for Modeling Multi-Site Count Outcomes in Distributed Data Networks. Journal of Biomedical Informatics, (2022): 104097.
- Luo, CL, Duan, R, Naj, A, Kranzler, H, Bian, J and Chen, Y. A One-shot Distributed Algorithm for Cox model with Heterogeneous Multicenter Data. Scientific Reports, 12.1 (2022): 1-8.
- Luo, C., Islam, M.N., Sheils, N.E., Buresh, J., Reps, J.M., Martijn, S, Ryan, P, Edmondson, M., Duan, R., Tong, J., Marks-Anglin, A, Bian, J, Chen, Z, Duarte- Salles, T, Fernandez-Bertolin, S, Falconer, T, Kim, C, Park, RW, Pfohl, S, Shah, Nigam, Williams, A, Xu, H, Zhou, Y, Lautenbach, E, Doshi, J, Werner, R, Asch, D and Chen, Y. (February 2022) DLMM as a lossless one-shot algorithm for collaborative multi-site distributed linear mixed models. Nature Communications, 13.1 (2022): 1-10.
- Liu, XK, Chubak, J, Hubbard, R and Chen, Y. SAT: a Surrogate Assisted Two- wave case boosting sampling method, with application to EHR-based association studies. Journal of the American Medical Informatics Association, Volume 29, Issue 5, May 2022, Pages 918–927.
- Yin, Z, Tong, J, Chen, Y, Hubbard, R, and Tang, CY. A Cost-effective Chart Review Sampling Design to Account for Phenotyping Error in EHR data. Journal of the American Medical Informatics Association, (2021) 29(1):52-61.
- Edmondson, M, Luo, C, Duan, R, Maltenfort, M, Chen, Z, Locke, K, Shults, J, Bian, J, Ryan, P, Forrest, C and Chen, Y. An Efficient and Accurate Distributed Learning Algorithm for Modeling Multi-Site Zero-Inflated Count Outcomes. Scientific Reports 11.1 (2021): 1-17.
- Du, J, Xiang, Y, Sankaranarayanapillai, M, Zhang, M, Wang, J, Si, Y, Pham, H, Xu, H, Chen, Y, Tao, C. Extracting post-marketing adverse events from safety reports in the Vaccine Adverse Event Reporting System (VAERS) using deep learning, (2021) Journal of the American Medical Informatics Association, 28.7 (2021): 1393-1400.
- Hubbard, R, Xu, J, Siegel, R, Chen, Y, Ihuoma Eneli. Studying pediatric health outcomes with electronic health records using Bayesian clustering and trajectory analysis, (December 2020) Journal of Biomedical Informatics, 113 (2021): 103654.
- Li, R, Duan, R, Zhang, X, Lumley, T, Pendergrass, S, Bauer, C, Hakonarson, H, Carrell, D, Smoller, J, Wei, W, Carroll, R, Edwards, D, Wiesner, G, Sleiman, P, Denny, J, Mosley, J, Ritchie, M, Chen, Y and Moore, J. Lossless integration of multiple electronic health records for identifying pleiotropy using summary statistics. (October 2020) Nature Communications, 12.1 (2021): 1-10. selected as Best paper award by the Translational Bioinformatics Year-in-Review by American Medical Informatics Association (top 25 papers among 206 papers published in Jan 2020 – March 2021)
- Raisaro, JL, Marino, F, Troncoso-Pastoriza, J, Bernstam, E.V., Chen, Y, Got- tlieb, A, Kim, M, Klann, J, Klersy, C, Malin, B, Mean, M, Prasser, F, Scudeller, L, Wong, S, Frenkel-Morgenstern, M, Xu, H, Jiang, X, Hubaux, JP. (July 2020) SCOR: A secure international informatics infrastructure to investigate COVID-19. Journal of the American Medical Informatics Association, 27.11 (2020): 1721-1726.
- Duan, R, Luo, C, Schuemie, M.J., Tong, J, Liang, J., Chang, H., Boland, M.R., Bian, J., Xu, H., Holmes, J., Forrest, C., Morton, S., Berlin, J.A., Moore, J.H., Mahoney, K.B. and Chen, Y. (June 2020) Learning from local to global – an efficient distributed algorithm for modeling time to event data, Journal of the American Medical Informatics Association 27.7 (2020): 1028-1036.
- Duan, R, Boland, MB, Liu, ZX, Liu, Y, Chang, H, Xu, H, Chu, H, Schmid, C, For- rest, C, Holmes, J, Schuemie, M, Berlin, J.A. and Chen, Y. (Oct 2019) Learning from Electronic Health Records Across Multiple Sites: A Communication-efficient and Privacy-preserving Distributed Algorithm. Journal of the American Medical Informatics Association 27.3 (2020): 376-385.
- Tong, J, Huang, J, Wang, X, Moore, J, Hubbard, R and Chen, Y. (Sep. 2019) An Augmented Estimation Procedure for EHR-based Association Studies Accounting for Differential Misclassification. Journal of the American Medical Informatics Association 27.2 (2020): 244-253.
- Du, J, Cunningham, RM, Xiang, Y, Li, F, Jia, Y, Boom, JA, Myneni, S, Bian, J, Luo, C, Chen, Y and Tao, C. Leveraging deep learning to understand health beliefs about the Human Papillomavirus Vaccine from social media. Nature Partner Journal (NPJ) Digital Medicine, 2.1 (2019): 1-4.
- Li, R, Duan, R, Kember, R, Regeneron Genetic Center, Rader, D, Damrauer, S, Moore, J and Chen, Y. A regression framework to uncover pleiotropy in large-scale electronic health record data. Journal of the American Medical Informatics Association 26.10 (2019): 1083-1090.
- Li, R, Chen, Y, and Moore, J Integration of genetic and clinical information to improve imputation of data missing from electronic health records. Journal of the American Medical Informatics Association 26.10 (2019): 1056-1063.
- Huang, J, Duan, R, Hubbard, R, Wu, Y, Moore, JH, Xu, H, and Chen, Y, PIE: A prior knowledge guided integrated likelihood estimation method (PIE) for bias reduction in association studies using electronic health records data, Journal of the American Medical Informatics Association. 25.3 (2018): 345-352.
- Selected Epidemiology and Biomedical Science Papers:
- Zhou, T, Zhou, J, Hodges, J, Lin, L, Chen, Y, Cole, S, and Chu, H, Estimating the Complier Average Causal Effect in a Meta-analysis of Randomized Clinical Trials with Binary Outcomes Accounting for Noncompliance: A Generalized Linear Latent and Mixed Model Approach, American Journal of Epidemiology 191.1 (2022): 220-229.
- Xiao, M, Chen, Y, Cole, S, MacLehose RF, Richardson, DB, Chu, H. Is OR “portable” in meta-analysis? Time to consider bivariate generalized linear mixed model, Journal of Clinical Epidemiology 142 (2022): 280.
- Rao, S, Lee, G, Razzaghi, H, Lorman, V, Mejias, A, Pajor, N, Thacker, D, Webb, R, Dickinson, K, Bailey, C, Jhaveri, R, Christakis, D, Bennett, T, Chen, Y, and Forrest, C. Clinical Features and Burden of Postacute Sequelae of SARS-CoV-2 Infection in Children and Adolescents. JAMA Pediatrics, 176.10 (2022): 1000-1009.
- Lu, Y, Zandt, M, Liu, Y, Li, J, Wang, X, Chen, Y, Cho, J, Dorajoo, S, Feng, M, Hsu, M, Hsu, J, Iqbal, U, Chen, J, Jonnagaddala, J, Li, Y, Liaw, S, Lim, H, Ngiam, KY, Nguyen, P, Park, R, Pratt, N, Reich, C, Rhee, S, Sathappan, S, Shin, S, Tan, H, You, S, Zhang, X, Krumholz, H, Suchard, M, Xu, H. Analysis of dual combination therapies used in treatment of hypertension in a multinational cohort. JAMA Network Open, 5.3 (2022): e223877-e223877.
- Asch, D, Islam, M, Scheils, N, Chen, Y, Doshi, J, Buresh, J. and Werner, R. Patient and Hospital Factors Associated With Differences in Mortality Rates Among Black and White US Medicare Beneficiaries Hospitalized With COVID-19 Infection. JAMA Network Open, 4.6 (2021): e2112842-e2112842.
- Asch, D, Scheils, N, Islam, M, Chen, Y, Werner, R, Buresh, J and Doshi, J. Variation in US hospital mortality rates for patients admitted with COVID-19 during the first 6 months of the pandemic. JAMA Internal Medicine, 181.4 (2021): 471-478.
- Xiao, M, Chu, H, Cole, S, Chen, Y, MacLehose, R, Richardson, DB and Green- land, S. Odds ratios are far from “portable”—A call to use realistic models for effect variation in meta-analysis, Journal of Clinical Epidemiology (August 2021) (accepted).
- Hong, C, Duan, R, Zeng, L, Hubbard, R, Lumley, T, Riley, R, Chu, H, Kimmel, S and Chen, Y. The Galaxy plot: a new visualization tool of bivariate meta-analysis studies, American Journal of Epidemiology 189.8 (2020): 861-869.
- Du, J, Luo, C, Shegog, R, Bian, J, Cunningham, R, Boom, J, Poland, G, Chen, Y, Tao, C. Use of deep learning to analyze Twitter discussions about HPV vaccine beliefs and attitudes, JAMA Network Open 3.11 (2020): e2022025-e2022025.
- Singh, J, Kallan, M, Chen, Y, Parks, M, Ibrahim, S. Association of Race/Ethnicity with Hospital discharge disposition after Elective Total Knee Arthroplasty, JAMA Network Open, 2.10 (2019): e1914259-e1914259.
- Wang, L, Rouse, B, Marks-Anglin, A, Duan, R, Shi, Q, Quach, K, Chen, Y Schmid, CH, Li, T. Rapid network meta-analysis using data from Food and Drug Administration approval packages is feasible but with limitations. Journal of Clinical Epidemiology. 114:84–94.
- Chahoud, J, Semaan, A, Chen, Y, Cao, M, Rieber, A, Rady, P and Tyring, S. The Association between Beta-genus Human Papillomavirus and Cutaneous Squamous Cell Carcinoma in Immunocompetent Individuals: a Meta-analysis, JAMA Dermatology, 152(12):1354–1364.
- Gluck, C, Qiu, C, Han, S, Palmer, M, Park, J, Ko, Y, Hanson, R, Huang, J, Chen, Y, Park, A, Mantzaris, I, Verma, A, Li, H, and Susztak, K. Kidney cytosine methylation changes improve renal function decline estimation in patients with diabetic kidney disease, Nature Communications, 10.1 (2019): 1-12.
- Lake, E, Jordan, J, Duan, R, and Chen, Y. A Meta-Analysis of the Associations Between the Nurse Work Environment in Hospitals and 4 Sets of Outcomes, Medical Care 59(5) 353-360.12
Teaching
Courses Taught at the University of Pennsylvania
Course instructors:
Yong Chen
Description:
This graduate-level Biostatistics course will introduce the fundamentals of statistical methods for meta-analyses. It will cover key principles of meta-analysis and the statistical rationales behind the analytic models, including univariate meta-analysis, multivariate meta-analysis, meta-analysis of diagnostic test accuracy, network meta-analysis, and multivariate network meta-analysis. Beyond these commonly used models, the course will cover statistical methods and software that investigate and correct for biases in systematic reviews such as publication bias, outcome reporting bias. Advanced statistical inferential tools such as composite likelihood, pseudolikelihood, integrated likelihood methods, EM algorithms will be introduced.
In addition, the cover will also cover some practical steps in systematic review including search strategies, data abstraction methods; quality assessment; and writing a meta-analysis report.
The course is composed of a series of weekly lectures and small group discussions. Students will be expected to attend weekly lectures, participate in class discussions, review assigned readings, complete homework assignments, and conduct a real-world meta-analysis with a clinically meaningful problem.
The students will be evaluated based on 2 homework assignments and a final in-class presentation of their final projects.
Textbooks:
1. [Primary textbook] Schwarzer, Guido, Carpenter, James R., Rücker, Gerta. Meta-Analysis with R. Springer 2015.
2. [Primary textbook] Egger, Matthias, George D. Smith, and Douglas G. Altman, eds. Systematic Reviews in Health Care: Meta-analysis in Context. London: BMJ Publishing Group, 2001.
3. [Optional textbook] Borenstein, Michael, Larry V. Hedges, Julian P. T. Higgins, Hannah R. Rothstein. Introduction to Meta-Analysis. Wiley, 2009.
4. [Optional textbook] Rothstein, Hannah R., Alexander J. Sutton, Michael Borenstein. Publication Bias in Meta-Analysis: Prevention, Assessment and Adjustments. Wiley, 2005.
Course format:
This course will have a hybrid lecture/seminar format, with Dr. Yong Chen presenting lectures on standard and advanced statistical methods for meta-analysis, and several guests who will describe important aspects of systematic review from their perspectives as clinicians, epidemiologists, medical librarians, and systematic reviewers. The guest speakers include Drs. Jesse Berlin (Johnson & Johnson Ltd), Robert J. DeRubeis (UPenn), Eileen Erinoff (Emergency Care Research Institute, ECRI), Tianjing Li (the Johns Hopkins University School of Public Health). All of them have given guest lectures co-directed by Dr. Yong Chen two years ago.
Expectation:
This course is expected to attract students from the first year and above in their PhD program, and will likely include students in GGEB (Biostatistics and Epidemiology programs) as well as perhaps students in other groups, such as MSCE students, who meet the prerequisites.
Course instructors:
Justine Shults (part I: Linear models) and Yong Chen (Part II: Generalized linear models)
Description:
This is a course on methods for generalized linear models (GLMs), rather than a course on using software for data analysis with GLMs. This course is designed to provide students with a fundamental understanding of theory and applications of the GLMs. Emphasis will be placed on statistical modeling, building from standard normal linear models, extending to GLMs, and going beyond GLMs. The main subjects are logit models for nominal and ordinal data, log-linear models, models for repeated categorical data, generalized linear mixed models and other mixture models for categorical data. Methods of maximum likelihood, weighted least squares, and generalized estimating equations will be used for estimation and inference.”
Textbooks:
1. Agresti, A. (2002). Categorical Data Analysis (Second Edition). Wiley. ISBN-10: 0471360937.
2. McCullagh, P. and Nelder, J.A. (1989) Generalized Linear Models (Second Edition). Chapman and Hall. ISBN-10: 0412317605.
Learning objectives:
Regression analysis has been developed for many years and remains one of the most commonly used statistical tools to help scientists address their scientific questions. Generalized linear models (GLMs) were formulated by John Nelder and Robert Wedderburn as a way of unifying various other statistical models, including ANCOVA, linear regression, logistic regression and log-linear models for contingency tables and count data. This lecture will introduce GLMs and some recent developments of regression techniques with focus on generalized linear models, quasi likelihood methods and estimating function approaches.
List of topics:
-
Generalized linear models and maximum likelihood method
-
Quasi-likelihood method and estimating equation
-
Model selection
-
Analysis of binary data
-
Analysis of polytomous responses
-
Analysis of count data: log linear models
-
Analysis of contingency table
-
Generalized linear mixed effect models
-
Analysis of matched data
-
Inference for correlated responses: marginal models and random effect models
Expectation:
By the end of the course, the students are expected to: 1) understand the main components of GLMs; 2) build and apply appropriate models to binary, nominal, ordinal or count data; 3) build and apply appropriate models to correlated outcomes; 4) make inference for a given model and interpret the results in the scientific context
Course directors:
Craig Umscheid and Yong Chen
Objective:
This 1.0 unit graduate-level course will provide an introduction to the fundamentals of systematic reviews and meta-analyses. It will cover introductory principles of meta-analysis; protocol development; search strategies; data abstraction methods; quality assessment; meta-analytic methods; and applications of meta-analysis. The course is composed of a series of weekly small group lectures and discussions. Students will be expected to attend weekly didactics, participate in class discussions, review assigned readings, complete homework assignments, and draft a systematic review protocol of their choosing suitable for IRB submission.
Assignments:
Students will be required to complete readings in the textbook and articles referenced for each session. In addition, each student will complete homework assignments assigned by the instructors including a data analysis project using a meta-analysis dataset provided by the instructors: download Stata meta-analysis modules from the Stata website, review dataset variables, complete an analysis, and write-up their findings. Finally, students will draft a systematic review protocol of their choosing and present their protocol at the conclusion of the class. There are no examinations.
Course instructors:
Yong Chen (Part I) and Jinbo Chen (Part II)
Outline of topics:
Parametric Inference:
Unbiased estimation and unbiased estimating functions
Maximum likelihood estimation: Consistency, asymptotic normality, and efficiency
Hypothesis testing: Wald test, Likelihood ratio test, Score test
Influence functions
EM algorithm
Model checking, Model mis-specification, and model selection
Examples of Non-regular maximum likelihood estimation
Marginal likelihood, Conditional likelihood, (modified) profile likelihood, composite likelihood, and pseudolikelihood
U-statistics theory
Contiguity theory
Bayes and Empirical Bayes estimators, Bayesian tests
Semiparametric Inference:
Semiparametric maximum likelihood estimation (Case-control study; Cox proportional hazards regression)
Z-estimation/M-estimation
Generalized score test, with Pearson’s Chi^2 test as an example
Semiparametric inference with incomplete data
Course instructors:
Yong Chen
Description:
This course presents extensions of general and generalized linear models to longitudinal and correlated outcome data with special emphasis on clinical, epidemiologic, and public health applications. Major topics include generalized linear mixed linear models (GLMM) for continuous, binomial, and count data; maximum likelihood estimation; generalized estimating equations (GEE); current general and specialized software applicable to these methods; and readings from current statistical literature. Each student will be required to participate in 4 labs and complete associated problem sets. Software will include Stata.
Textbooks:
1. Diggle, P, Heagerty, P, Liang, K-Y and Zeger, S. (2013). Analysis of Longitudinal Data (Second Edition). Oxford University Press. ISBN-10: 0198524846.
2. Fitzmaurice GM, Laird NM, Ware JH. Applied Longitudinal Analysis. Second Edition. New York: Wiley; 2011. ISBN: 978-0-470-38027-7. Hardcover 740 pages; August 2011
3. Singer JD, Willett JB. Applied Longitudinal Analysis. New York: Oxford 2003.
Graphics texts:
Mitchell MN. A Visual Guide to Stata Graphics. 3rd Edition. College Station, TX: Stata Press; 2012.
Courses Taught at the University of Texas School of Public Health
Course instructor:
Yong Chen
Description:
This is a course on methods for generalized linear models (GLMs), rather than a course on using software for data analysis with GLMs. This course is designed to provide students with a fundamental understanding of theory and applications of the GLMs. Emphasis will be placed on statistical modeling, building from standard normal linear models, extending to GLMs, and going beyond GLMs. The main subjects are logit models for nominal and ordinal data, log-linear models, models for repeated categorical data, generalized linear mixed models and other mixture models for categorical data. Methods of maximum likelihood, weighted least squares, and generalized estimating equations will be used for estimation and inference.”
Textbooks:
1. Agresti, A. (2002). Categorical Data Analysis (Second Edition). Wiley. ISBN-10: 0471360937.
2. McCullagh, P. and Nelder, J.A. (1989) Generalized Linear Models (Second Edition). Chapman and Hall. ISBN-10: 0412317605.
Learning objectives:
Regression analysis has been developed for many years and remains one of the most commonly used statistical tools to help scientists address their scientific questions. Generalized linear models (GLMs) were formulated by John Nelder and Robert Wedderburn as a way of unifying various other statistical models, including ANCOVA, linear regression, logistic regression and log-linear models for contingency tables and count data. This lecture will introduce GLMs and some recent developments of regression techniques with focus on generalized linear models, quasi likelihood methods and estimating function approaches.
List of topics:
-
Generalized linear models and maximum likelihood method
-
Quasi-likelihood method and estimating equation
-
Model selection
-
Analysis of binary data
-
Analysis of polytomous responses
-
Analysis of count data: log linear models
-
Analysis of contingency table
-
Generalized linear mixed effect models
-
Analysis of matched data
-
Inference for correlated responses: marginal models and random effect models
Expectation:
By the end of the course, the students are expected to: 1) understand the main components of GLMs; 2) build and apply appropriate models to binary, nominal, ordinal or count data; 3) build and apply appropriate models to correlated outcomes; 4) make inference for a given model and interpret the results in the scientific context.
Course instructors:
Yong Chen
Description:
This course presents extensions of general and generalized linear models to longitudinal and correlated outcome data with special emphasis on clinical, epidemiologic, and public health applications. Major topics include generalized linear mixed linear models (GLMM) for continuous, binomial, and count data; maximum likelihood estimation; generalized estimating equations (GEE); current general and specialized software applicable to these methods; and readings from current statistical literature. Each student will be required to participate in 4 labs and complete associated problem sets. Software will include Stata.
Textbooks:
1. Diggle, P, Heagerty, P, Liang, K-Y and Zeger, S. (2013). Analysis of Longitudinal Data (Second Edition). Oxford University Press. ISBN-10: 0198524846.
2. Fitzmaurice GM, Laird NM, Ware JH. Applied Longitudinal Analysis. Second Edition. New York: Wiley; 2011. ISBN: 978-0-470-38027-7. Hardcover 740 pages; August 2011
3. Singer JD, Willett JB. Applied Longitudinal Analysis. New York: Oxford 2003.
Graphics texts:
Mitchell MN. A Visual Guide to Stata Graphics. 3rd Edition. College Station, TX: Stata Press; 2012.
Course instructor:
Yong Chen
Description:
This is a course on methods for generalized linear models (GLMs), rather than a course on using software for data analysis with GLMs. This course is designed to provide students with a fundamental understanding of theory and applications of the GLMs. Emphasis will be placed on statistical modeling, building from standard normal linear models, extending to GLMs, and going beyond GLMs. The main subjects are logit models for nominal and ordinal data, log-linear models, models for repeated categorical data, generalized linear mixed models and other mixture models for categorical data. Methods of maximum likelihood, weighted least squares, and generalized estimating equations will be used for estimation and inference.”
Textbooks:
1. Agresti, A. (2002). Categorical Data Analysis (Second Edition). Wiley. ISBN-10: 0471360937.
2. McCullagh, P. and Nelder, J.A. (1989) Generalized Linear Models (Second Edition). Chapman and Hall. ISBN-10: 0412317605.
Learning objectives:
Regression analysis has been developed for many years and remains one of the most commonly used statistical tools to help scientists address their scientific questions. Generalized linear models (GLMs) were formulated by John Nelder and Robert Wedderburn as a way of unifying various other statistical models, including ANCOVA, linear regression, logistic regression and log-linear models for contingency tables and count data. This lecture will introduce GLMs and some recent developments of regression techniques with focus on generalized linear models, quasi likelihood methods and estimating function approaches.
List of topics:
-
Generalized linear models and maximum likelihood method
-
Quasi-likelihood method and estimating equation
-
Model selection
-
Analysis of binary data
-
Analysis of polytomous responses
-
Analysis of count data: log linear models
-
Analysis of contingency table
-
Generalized linear mixed effect models
-
Analysis of matched data
-
Inference for correlated responses: marginal models and random effect models
Expectation:
By the end of the course, the students are expected to: 1) understand the main components of GLMs; 2) build and apply appropriate models to binary, nominal, ordinal or count data; 3) build and apply appropriate models to correlated outcomes; 4) make inference for a given model and interpret the results in the scientific context.
Course instructor:
Yong Chen
Description:
This is a course on methods for generalized linear models (GLMs), rather than a course on using software for data analysis with GLMs. This course is designed to provide students with a fundamental understanding of theory and applications of the GLMs. Emphasis will be placed on statistical modeling, building from standard normal linear models, extending to GLMs, and going beyond GLMs. The main subjects are logit models for nominal and ordinal data, log-linear models, models for repeated categorical data, generalized linear mixed models and other mixture models for categorical data. Methods of maximum likelihood, weighted least squares, and generalized estimating equations will be used for estimation and inference.”
Textbooks:
1. Agresti, A. (2002). Categorical Data Analysis (Second Edition). Wiley. ISBN-10: 0471360937.
2. McCullagh, P. and Nelder, J.A. (1989) Generalized Linear Models (Second Edition). Chapman and Hall. ISBN-10: 0412317605.
Learning objectives:
Regression analysis has been developed for many years and remains one of the most commonly used statistical tools to help scientists address their scientific questions. Generalized linear models (GLMs) were formulated by John Nelder and Robert Wedderburn as a way of unifying various other statistical models, including ANCOVA, linear regression, logistic regression and log-linear models for contingency tables and count data. This lecture will introduce GLMs and some recent developments of regression techniques with focus on generalized linear models, quasi likelihood methods and estimating function approaches.
List of topics:
-
Generalized linear models and maximum likelihood method
-
Quasi-likelihood method and estimating equation
-
Model selection
-
Analysis of binary data
-
Analysis of polytomous responses
-
Analysis of count data: log linear models
-
Analysis of contingency table
-
Generalized linear mixed effect models
-
Analysis of matched data
-
Inference for correlated responses: marginal models and random effect models
Expectation:
By the end of the course, the students are expected to: 1) understand the main components of GLMs; 2) build and apply appropriate models to binary, nominal, ordinal or count data; 3) build and apply appropriate models to correlated outcomes; 4) make inference for a given model and interpret the results in the scientific context.
Course instructors:
Yong Chen
Description:
This course presents extensions of general and generalized linear models to longitudinal and correlated outcome data with special emphasis on clinical, epidemiologic, and public health applications. Major topics include generalized linear mixed linear models (GLMM) for continuous, binomial, and count data; maximum likelihood estimation; generalized estimating equations (GEE); current general and specialized software applicable to these methods; and readings from current statistical literature. Each student will be required to participate in 4 labs and complete associated problem sets. Software will include Stata.
Textbooks:
1. Diggle, P, Heagerty, P, Liang, K-Y and Zeger, S. (2013). Analysis of Longitudinal Data (Second Edition). Oxford University Press. ISBN-10: 0198524846.
2. Fitzmaurice GM, Laird NM, Ware JH. Applied Longitudinal Analysis. Second Edition. New York: Wiley; 2011. ISBN: 978-0-470-38027-7. Hardcover 740 pages; August 2011
3. Singer JD, Willett JB. Applied Longitudinal Analysis. New York: Oxford 2003.
Graphics texts:
Mitchell MN. A Visual Guide to Stata Graphics. 3rd Edition. College Station, TX: Stata Press; 2012.
Course instructor:
Yong Chen
Description:
This is a course on methods for generalized linear models (GLMs), rather than a course on using software for data analysis with GLMs. This course is designed to provide students with a fundamental understanding of theory and applications of the GLMs. Emphasis will be placed on statistical modeling, building from standard normal linear models, extending to GLMs, and going beyond GLMs. The main subjects are logit models for nominal and ordinal data, log-linear models, models for repeated categorical data, generalized linear mixed models and other mixture models for categorical data. Methods of maximum likelihood, weighted least squares, and generalized estimating equations will be used for estimation and inference.”
Textbooks:
1. Agresti, A. (2002). Categorical Data Analysis (Second Edition). Wiley. ISBN-10: 0471360937.
2. McCullagh, P. and Nelder, J.A. (1989) Generalized Linear Models (Second Edition). Chapman and Hall. ISBN-10: 0412317605.
Learning objectives:
Regression analysis has been developed for many years and remains one of the most commonly used statistical tools to help scientists address their scientific questions. Generalized linear models (GLMs) were formulated by John Nelder and Robert Wedderburn as a way of unifying various other statistical models, including ANCOVA, linear regression, logistic regression and log-linear models for contingency tables and count data. This lecture will introduce GLMs and some recent developments of regression techniques with focus on generalized linear models, quasi likelihood methods and estimating function approaches.
List of topics:
-
Generalized linear models and maximum likelihood method
-
Quasi-likelihood method and estimating equation
-
Model selection
-
Analysis of binary data
-
Analysis of polytomous responses
-
Analysis of count data: log linear models
-
Analysis of contingency table
-
Generalized linear mixed effect models
-
Analysis of matched data
-
Inference for correlated responses: marginal models and random effect models
Expectation:
By the end of the course, the students are expected to: 1) understand the main components of GLMs; 2) build and apply appropriate models to binary, nominal, ordinal or count data; 3) build and apply appropriate models to correlated outcomes; 4) make inference for a given model and interpret the results in the scientific context.
Joint appointments:
- Senior Fellow at the Institute of Biomedical Informatics, University of Pennsylvania
- Senior Scholar at the Center for Evidence-based Practice at Penn School of Medicine, University of Pennsylvania
- Faculty member at the Applied Mathematics & Computational Science Program, Penn Arts & Sciences, University of Pennsylvania