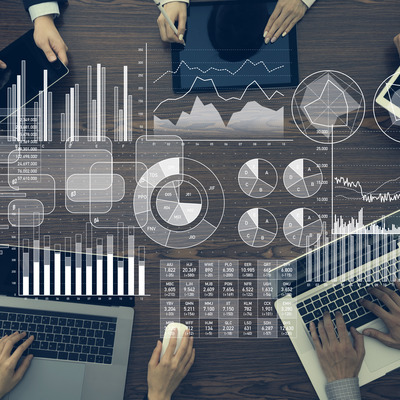
Statistical inference
We have developed general theory for statistical inference for situations with non-standard problems that arise in applications including correlated data, variance component models, multivariate survival models, and mixture models. When these non-regular problems occur, special attention is needed to design test statistics that overcome misspecification of Type I error and substantial loss of statistical power. In a sequence of papers, published at Biometrika, one of the most prestigious journals in statistics, we laid a foundation of rigorous statistical inference on these non-regular problems (Chen and Liang, 2010, Biometrika; Chen et al., 2017, Biometrika; Chen et al, 2018, Biometrika). We have used the theoretical framework to develop inferential tools to develop tests for homogeneity in mixture models that are relevant to analysis of gene expression data, DNA methylation data, and genetic quantitative trait locus analyses (Chen et al., 2013, Genetic Epidemiology; Ning and Chen, 2015, Scandinavian Journal of Statistics; Hong et al., 2016, JASA; Hong et al., 2016, Biometrics), and pharmacovigilance studies (Cai et al, 2016, BMC Medical Informatics and Decision Making; Huang et al., 2018, Statistica Sinica).
We have also contributed to methods for longitudinal data analysis and multivariate survival analysis. Motivated by a study on evaluating the effectiveness of an intervention for weight loss using self-reported weights, we developed a novel framework of pseudo-likelihood methods to analyze longitudinal data where the observation times may be outcome dependent, in which case the standard generalized estimating equation (GEE) approach fails (Chen et al., 2015, Biostatistics; Cai et al., 2018, Statistics in Medicine, under revision; Shen et al., 2017+, Statistica Sinica, under review). A key innovation of this framework is that, unlike joint modeling methods, the validity of this methods does not rely on the correct specification of the observation time process or the complex correlation structures, offering greater model robustness. Motivated from a soft tissue sarcoma study, we proposed a time-dependent measure and developed a pseudo-likelihood-based inference to quantify the local dependence between two types of recurrent event processes (e.g., local and distant disease recurrences), without specifying the joint recurrent event processes (Ning et al., 2015, Biometrika). In addition, for analyzing re-offense data of juvenile probationers, he developed a frailty model for recurrent events during alternating restraint periods (e.g., placement in a community unit) and non-restraint periods (e.g., released to home), which corrects the bias induced by ignoring the differences between two periods, and leads to superior dynamic risk prediction (Li et al., 2016, Statistics in Medicine).