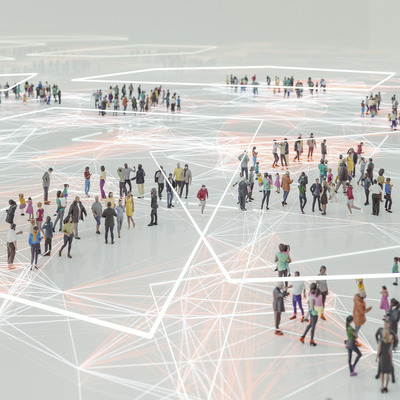
INTEGRATION OF HETEROGENEOUS GENETIC DATA
We have developed a novel meta-analytic framework for integrating data from multiple studies in identifying high-dimensional genetic risk factors of lung cancer and bladder cancer. We proposed a novel paradigm, YETI (phylogenY-aware Effect-size Tests for Interactions), for detecting genetic interactions from heterogeneous GWAS (Liu et al., 2016, Genetic Epidemiology); see the figure below.
We have recently applied this method to bladder cancer data (dbGaP) where information from the Spanish and Finnish populations (n=6,978) are integrated without sharing the raw data (Liu, et al., 2018, Genetic Epidemiology; under review), and have identified potential novel interactions; see the figure below.
We have also studied meta-analytical methods to quantify gene-environment interactions for lung cancer using four studies conducted in the State of Pennsylvania (n=1,610) (Huang et al., 2017, Genetic Epidemiology). The key innovations of this paradigm are to embrace heterogeneity across populations, and to combine information across studies without sharing raw data. These Big Data innovations are critical because (1) heterogeneity across datasets was first leveraged to improve statistical power, and (2) the algorithms were developed as distributed algorithms to avoid sharing raw data, which is critical for privacy protection.

We are currently collaborating with investigators at the Penn Institute for Translational Medicine and Therapeutics study a Penn biobank data. By linking EHR with genetic information, we are enabled to explore the contributions of genetic variations to multiple complex conditions. However, the high dimensional genetic data pose new challenges in statistical modeling and inference. This necessitates innovations. We are currently developing a novel statistical modeling and inferential framework to prioritize single nucleotide polymorphisms (SNP)s for identifying pleiotropic effects.